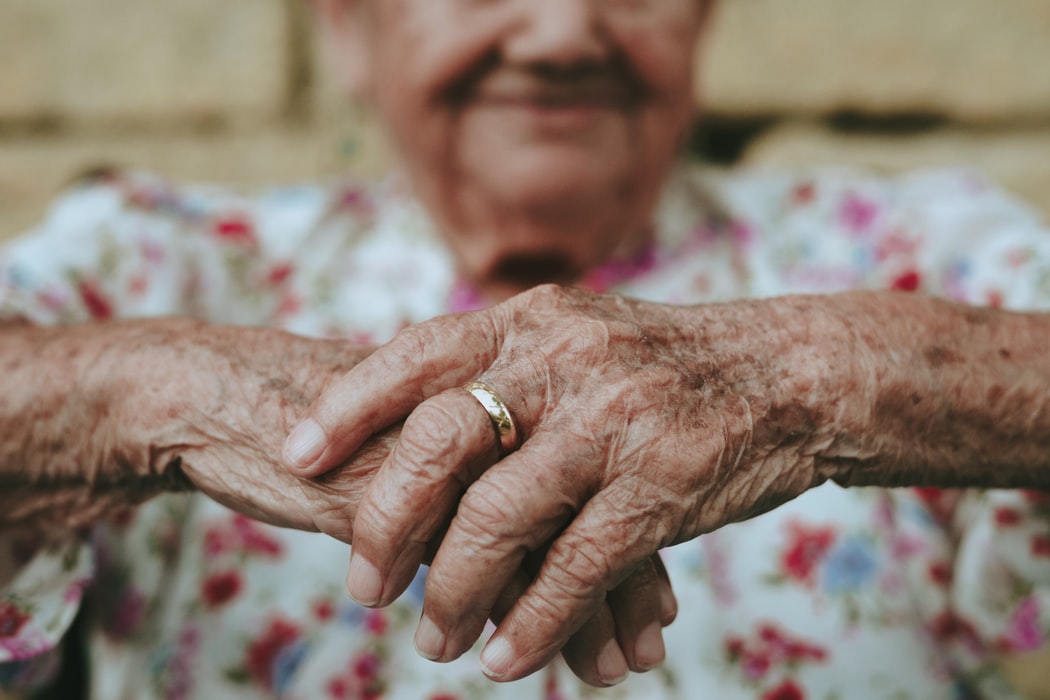
Device-measured sedentary behavior in oldest old adults: A systematic review and meta-analysis
September 7, 2021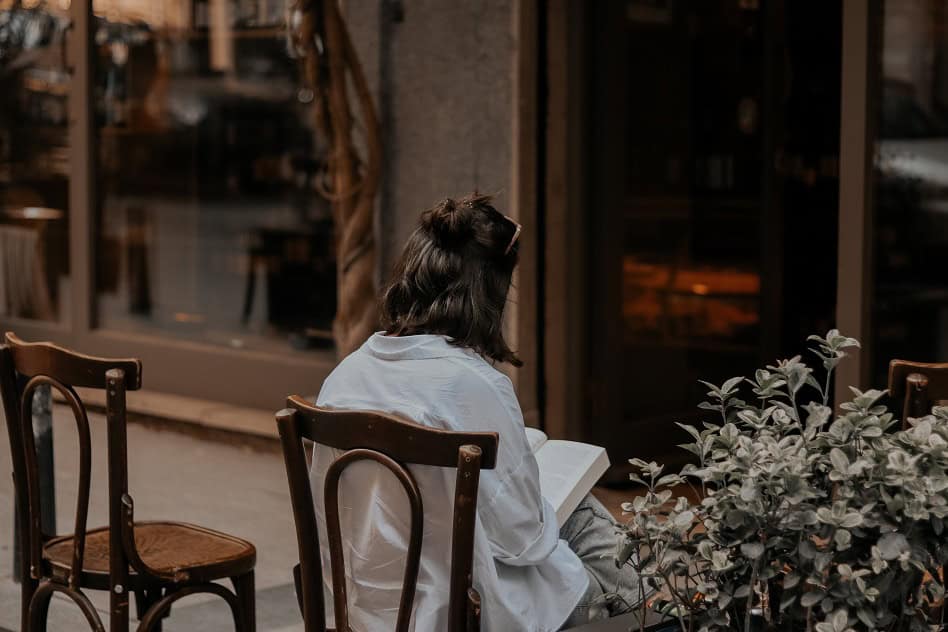
Efficacy, characteristics, behavioural models and behaviour change strategies, of non-workplace interventions specifically targeting sedentary behaviour; a systematic review and meta-analysis of randomised control trials in healthy ambulatory adults
September 21, 2021A paper titled “Application of Convolutional Neural Network Algorithms for Advancing Sedentary and Activity Bout Classification” has recently been published in the Journal for the Measurement of Physical Behaviour. Citation details and the summary of the paper are re-posted below. The full publication is available here (open access).
Citation
Nakandala, S., Jankowska, M. M., Tuz-Zahra, F., Bellettiere, J., Carlson, J. A., LaCroix, A. Z., Hartman, S. J., Rosenberg, D. E., Zou, J., Kumar, A., & Natarajan, L. (2021). Application of Convolutional Neural Network Algorithms for Advancing Sedentary and Activity Bout Classification. Journal for the Measurement of Physical Behaviour, 4(2), 102–110. https://doi.org/10.1123/jmpb.2020-0016
Abstract
Background: Machine learning has been used for classification of physical behavior bouts from hip-worn accelerometers; however, this research has been limited due to the challenges of directly observing and coding human behavior “in the wild.” Deep learning algorithms, such as convolutional neural networks (CNNs), may offer better representation of data than other machine learning algorithms without the need for engineered features and may be better suited to dealing with free-living data. The purpose of this study was to develop a modeling pipeline for evaluation of a CNN model on a free-living data set and compare CNN inputs and results with the commonly used machine learning random forest and logistic regression algorithms.
Method: Twenty-eight free-living women wore an ActiGraph GT3X+ accelerometer on their right hip for 7 days. A concurrently worn thigh-mounted activPAL device captured ground truth activity labels. The authors evaluated logistic regression, random forest, and CNN models for classifying sitting, standing, and stepping bouts. The authors also assessed the benefit of performing feature engineering for this task.
Results: The CNN classifier performed best (average balanced accuracy for bout classification of sitting, standing, and stepping was 84%) compared with the other methods (56% for logistic regression and 76% for random forest), even without performing any feature engineering.
Conclusion: Using the recent advancements in deep neural networks, the authors showed that a CNN model can outperform other methods even without feature engineering. This has important implications for both the model’s ability to deal with the complexity of free-living data and its potential transferability to new populations.