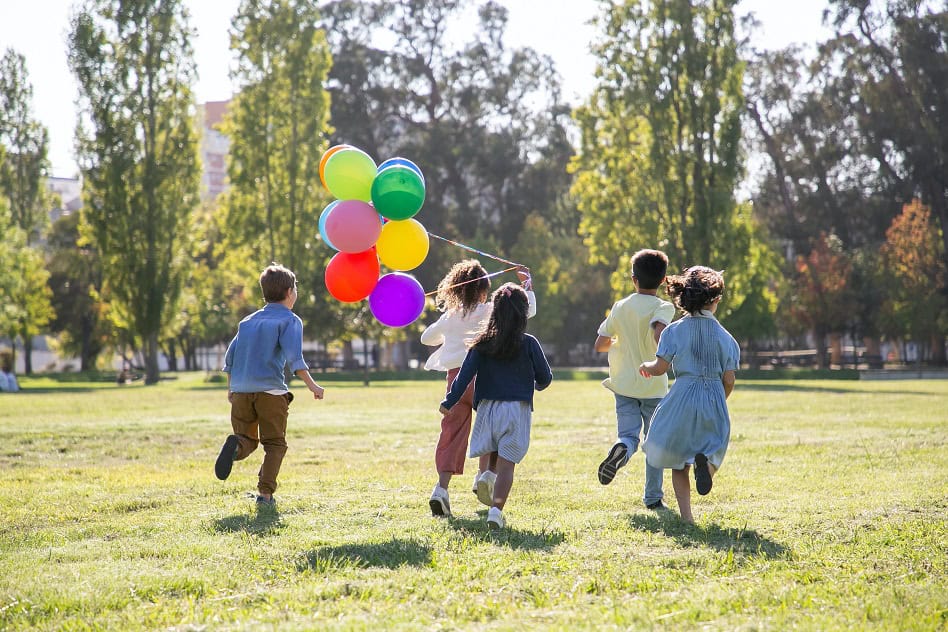
The Experimental Effect of Parent Versus Peer Influence on Children’s Physical Activity and Sedentary Behavior
February 9, 2022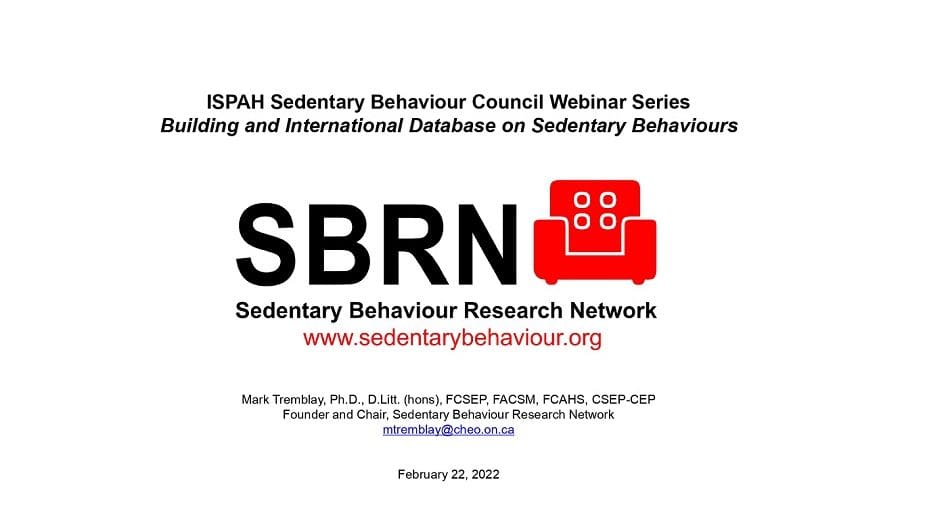
Professor Mark Tremblay presents at ISPAH Sedentary Behaviour Council webinar series
February 22, 2022A paper titled “Physical Activity, Sedentary Behavior, and Sleep on Twitter: Multicountry and Fully Labeled Public Data Set for Digital Public Health Surveillance Research” has recently been published in the JMIR Public Health & Surveillance. The full-text article is available here (open access). Citation details and the summary of the paper are re-posted below.
ABSTRACT
Background: Advances in automated data processing and machine learning (ML) models, together with the unprecedented growth in the number of social media users who publicly share and discuss health-related information, have made public health surveillance (PHS) one of the long-lasting social media applications. However, the existing PHS systems feeding on social media data have not been widely deployed in national surveillance systems, which appears to stem from the lack of practitioners and the public’s trust in social media data. More robust and reliable data sets over which supervised ML models can be trained and tested reliably is a significant step toward overcoming this hurdle. The health implications of daily behaviors (physical activity, sedentary behavior, and sleep [PASS]), as an evergreen topic in PHS, are widely studied through traditional data sources such as surveillance surveys and administrative databases, which are often several months out-of-date by the time they are used, costly to collect, and thus limited in quantity and coverage.
Objective: The main objective of this study is to present a large-scale, multicountry, longitudinal, and fully labeled data set to enable and support digital PASS surveillance research in PHS. To support high-quality surveillance research using our data set, we have conducted further analysis on the data set to supplement it with additional PHS-related metadata.
Methods: We collected the data of this study from Twitter using the Twitter livestream application programming interface between November 28, 2018, and June 19, 2020. To obtain PASS-related tweets for manual annotation, we iteratively used regular expressions, unsupervised natural language processing, domain-specific ontologies, and linguistic analysis. We used Amazon Mechanical Turk to label the collected data to self-reported PASS categories and implemented a quality control pipeline to monitor and manage the validity of crowd-generated labels. Moreover, we used ML, latent semantic analysis, linguistic analysis, and label inference analysis to validate the different components of the data set.
Results: LPHEADA (Labelled Digital Public Health Dataset) contains 366,405 crowd-generated labels (3 labels per tweet) for 122,135 PASS-related tweets that originated in Australia, Canada, the United Kingdom, or the United States, labeled by 708 unique annotators on Amazon Mechanical Turk. In addition to crowd-generated labels, LPHEADA provides details about the three critical components of any PHS system: place, time, and demographics (ie, gender and age range) associated with each tweet.
Conclusions: Publicly available data sets for digital PASS surveillance are usually isolated and only provide labels for small subsets of the data. We believe that the novelty and comprehensiveness of the data set provided in this study will help develop, evaluate, and deploy digital PASS surveillance systems. LPHEADA will be an invaluable resource for both public health researchers and practitioners.
CITATION
Shakeri Hossein Abad Z, Butler GP, Thompson W, Lee J. Physical Activity, Sedentary Behavior, and Sleep on Twitter: Multicountry and Fully Labeled Public Data Set for Digital Public Health Surveillance Research. JMIR Public Health Surveill. 2022;8(2):e32355. Published 2022 Feb 14. doi:10.2196/32355